Topic:Infrared And Visible Image Fusion
What is Infrared And Visible Image Fusion? Infrared-and-visible image fusion is the process of combining images from different spectral bands to enhance visual perception.
Papers and Code
Dec 18, 2024
Abstract:Infrared and visible image fusion (IVIF) is a crucial technique for enhancing visual performance by integrating unique information from different modalities into one fused image. Exiting methods pay more attention to conducting fusion with undisturbed data, while overlooking the impact of deliberate interference on the effectiveness of fusion results. To investigate the robustness of fusion models, in this paper, we propose a novel adversarial attack resilient network, called $\textrm{A}^{\textrm{2}}$RNet. Specifically, we develop an adversarial paradigm with an anti-attack loss function to implement adversarial attacks and training. It is constructed based on the intrinsic nature of IVIF and provide a robust foundation for future research advancements. We adopt a Unet as the pipeline with a transformer-based defensive refinement module (DRM) under this paradigm, which guarantees fused image quality in a robust coarse-to-fine manner. Compared to previous works, our method mitigates the adverse effects of adversarial perturbations, consistently maintaining high-fidelity fusion results. Furthermore, the performance of downstream tasks can also be well maintained under adversarial attacks. Code is available at https://github.com/lok-18/A2RNet.
* 9 pages, 8 figures, The 39th Annual AAAI Conference on Artificial
Intelligence
Via
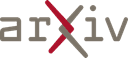
Dec 18, 2024
Abstract:Images captured in severe low-light circumstances often suffer from significant information absence. Existing singular modality image enhancement methods struggle to restore image regions lacking valid information. By leveraging light-impervious infrared images, visible and infrared image fusion methods have the potential to reveal information hidden in darkness. However, they primarily emphasize inter-modal complementation but neglect intra-modal enhancement, limiting the perceptual quality of output images. To address these limitations, we propose a novel task, dubbed visible and infrared information synthesis (VIIS), which aims to achieve both information enhancement and fusion of the two modalities. Given the difficulty in obtaining ground truth in the VIIS task, we design an information synthesis pretext task (ISPT) based on image augmentation. We employ a diffusion model as the framework and design a sparse attention-based dual-modalities residual (SADMR) conditioning mechanism to enhance information interaction between the two modalities. This mechanism enables features with prior knowledge from both modalities to adaptively and iteratively attend to each modality's information during the denoising process. Our extensive experiments demonstrate that our model qualitatively and quantitatively outperforms not only the state-of-the-art methods in relevant fields but also the newly designed baselines capable of both information enhancement and fusion. The code is available at https://github.com/Chenz418/VIIS.
* Accepted to WACV 2025
Via
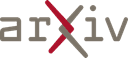
Dec 13, 2024
Abstract:Infrared and visible image fusion (IVIF) is a crucial technique for enhancing visual performance by integrating unique information from different modalities into one fused image. Exiting methods pay more attention to conducting fusion with undisturbed data, while overlooking the impact of deliberate interference on the effectiveness of fusion results. To investigate the robustness of fusion models, in this paper, we propose a novel adversarial attack resilient network, called $\textrm{A}^{\textrm{2}}$RNet. Specifically, we develop an adversarial paradigm with an anti-attack loss function to implement adversarial attacks and training. It is constructed based on the intrinsic nature of IVIF and provide a robust foundation for future research advancements. We adopt a Unet as the pipeline with a transformer-based defensive refinement module (DRM) under this paradigm, which guarantees fused image quality in a robust coarse-to-fine manner. Compared to previous works, our method mitigates the adverse effects of adversarial perturbations, consistently maintaining high-fidelity fusion results. Furthermore, the performance of downstream tasks can also be well maintained under adversarial attacks. Code is available at https://github.com/lok-18/A2RNet.
* 9 pages, 8 figures, The 39th Annual AAAI Conference on Artificial
Intelligence
Via
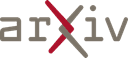
Dec 11, 2024
Abstract:The aim of multispectral image fusion is to combine object or scene features of images with different spectral characteristics to increase the perceptual quality. In this paper, we present a novel learning-based solution to image fusion problem focusing on infrared and visible spectrum images. The proposed solution utilizes only convolution and pooling layers together with a loss function using no-reference quality metrics. The analysis is performed qualitatively and quantitatively on various datasets. The results show better performance than state-of-the-art methods. Also, the size of our network enables real-time performance on embedded devices. Project codes can be found at \url{https://github.com/ferhatcan/pyFusionSR}.
* 2021 IEEE International Conference on Image Processing (ICIP),
pages 1779-1783, Publication date: 2021/9/19
* 5 pages, published at ICIP 2021
Via
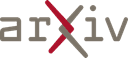
Dec 06, 2024
Abstract:Object detection in poor-illumination environments is a challenging task as objects are usually not clearly visible in RGB images. As infrared images provide additional clear edge information that complements RGB images, fusing RGB and infrared images has potential to enhance the detection ability in poor-illumination environments. However, existing works involving both visible and infrared images only focus on image fusion, instead of object detection. Moreover, they directly fuse the two kinds of image modalities, which ignores the mutual interference between them. To fuse the two modalities to maximize the advantages of cross-modality, we design a dual-enhancement-based cross-modality object detection network DEYOLO, in which semantic-spatial cross modality and novel bi-directional decoupled focus modules are designed to achieve the detection-centered mutual enhancement of RGB-infrared (RGB-IR). Specifically, a dual semantic enhancing channel weight assignment module (DECA) and a dual spatial enhancing pixel weight assignment module (DEPA) are firstly proposed to aggregate cross-modality information in the feature space to improve the feature representation ability, such that feature fusion can aim at the object detection task. Meanwhile, a dual-enhancement mechanism, including enhancements for two-modality fusion and single modality, is designed in both DECAand DEPAto reduce interference between the two kinds of image modalities. Then, a novel bi-directional decoupled focus is developed to enlarge the receptive field of the backbone network in different directions, which improves the representation quality of DEYOLO. Extensive experiments on M3FD and LLVIP show that our approach outperforms SOTA object detection algorithms by a clear margin. Our code is available at https://github.com/chips96/DEYOLO.
* The International Conference on Pattern Recognition (ICPR),2024
Via
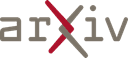
Dec 06, 2024
Abstract:Infrared (IR) imaging is commonly used in various scenarios, including autonomous driving, fire safety and defense applications. Thus, semantic segmentation of such images is of great interest. However, this task faces several challenges, including data scarcity, differing contrast and input channel number compared to natural images, and emergence of classes not represented in databases in certain scenarios, such as defense applications. Few-shot segmentation (FSS) provides a framework to overcome these issues by segmenting query images using a few labeled support samples. However, existing FSS models for IR images require paired visible RGB images, which is a major limitation since acquiring such paired data is difficult or impossible in some applications. In this work, we develop new strategies for FSS of IR images by using generative modeling and fusion techniques. To this end, we propose to synthesize auxiliary data to provide additional channel information to complement the limited contrast in the IR images, as well as IR data synthesis for data augmentation. Here, the former helps the FSS model to better capture the relationship between the support and query sets, while the latter addresses the issue of data scarcity. Finally, to further improve the former aspect, we propose a novel fusion ensemble module for integrating the two different modalities. Our methods are evaluated on different IR datasets, and improve upon the state-of-the-art (SOTA) FSS models.
* Winter Conference on Applications of Computer Vision (WACV), 2025
Via
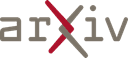
Nov 16, 2024
Abstract:Euclidean representation learning methods have achieved commendable results in image fusion tasks, which can be attributed to their clear advantages in handling with linear space. However, data collected from a realistic scene usually have a non-Euclidean structure, where Euclidean metric might be limited in representing the true data relationships, degrading fusion performance. To address this issue, a novel SPD (symmetric positive definite) manifold learning framework is proposed for multi-modal image fusion, named SPDFusion, which extends the image fusion approach from the Euclidean space to the SPD manifolds. Specifically, we encode images according to the Riemannian geometry to exploit their intrinsic statistical correlations, thereby aligning with human visual perception. Actually, the SPD matrix underpins our network learning, with a cross-modal fusion strategy employed to harness modality-specific dependencies and augment complementary information. Subsequently, an attention module is designed to process the learned weight matrix, facilitating the weighting of spatial global correlation semantics via SPD matrix multiplication. Based on this, we design an end-to-end fusion network based on cross-modal manifold learning. Extensive experiments on public datasets demonstrate that our framework exhibits superior performance compared to the current state-of-the-art methods.
* 14 pages, 12 figures
Via
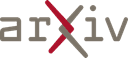
Nov 16, 2024
Abstract:Infrared and visible (IR-VIS) image fusion has gained significant attention for its broad application value. However, existing methods often neglect the complementary role of infrared image in restoring visible image features under hazy conditions. To address this, we propose a joint learning framework that utilizes infrared image for the restoration and fusion of hazy IR-VIS images. To mitigate the adverse effects of feature diversity between IR-VIS images, we introduce a prompt generation mechanism that regulates modality-specific feature incompatibility. This creates a prompt selection matrix from non-shared image information, followed by prompt embeddings generated from a prompt pool. These embeddings help generate candidate features for dehazing. We further design an infrared-assisted feature restoration mechanism that selects candidate features based on haze density, enabling simultaneous restoration and fusion within a single-stage framework. To enhance fusion quality, we construct a multi-stage prompt embedding fusion module that leverages feature supplementation from the prompt generation module. Our method effectively fuses IR-VIS images while removing haze, yielding clear, haze-free fusion results. In contrast to two-stage methods that dehaze and then fuse, our approach enables collaborative training in a single-stage framework, making the model relatively lightweight and suitable for practical deployment. Experimental results validate its effectiveness and demonstrate advantages over existing methods.
Via
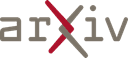
Nov 14, 2024
Abstract:The primary value of infrared and visible image fusion technology lies in applying the fusion results to downstream tasks. However, existing methods face challenges such as increased training complexity and significantly compromised performance of individual tasks when addressing multiple downstream tasks simultaneously. To tackle this, we propose Task-Oriented Adaptive Regulation (T-OAR), an adaptive mechanism specifically designed for multi-task environments. Additionally, we introduce the Task-related Dynamic Prompt Injection (T-DPI) module, which generates task-specific dynamic prompts from user-input text instructions and integrates them into target representations. This guides the feature extraction module to produce representations that are more closely aligned with the specific requirements of downstream tasks. By incorporating the T-DPI module into the T-OAR framework, our approach generates fusion images tailored to task-specific requirements without the need for separate training or task-specific weights. This not only reduces computational costs but also enhances adaptability and performance across multiple tasks. Experimental results show that our method excels in object detection, semantic segmentation, and salient object detection, demonstrating its strong adaptability, flexibility, and task specificity. This provides an efficient solution for image fusion in multi-task environments, highlighting the technology's potential across diverse applications.
* 10 pages, 7 figures
Via
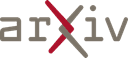
Dec 02, 2024
Abstract:RGB-Thermal Salient Object Detection aims to pinpoint prominent objects within aligned pairs of visible and thermal infrared images. Traditional encoder-decoder architectures, while designed for cross-modality feature interactions, may not have adequately considered the robustness against noise originating from defective modalities. Inspired by hierarchical human visual systems, we propose the ConTriNet, a robust Confluent Triple-Flow Network employing a Divide-and-Conquer strategy. Specifically, ConTriNet comprises three flows: two modality-specific flows explore cues from RGB and Thermal modalities, and a third modality-complementary flow integrates cues from both modalities. ConTriNet presents several notable advantages. It incorporates a Modality-induced Feature Modulator in the modality-shared union encoder to minimize inter-modality discrepancies and mitigate the impact of defective samples. Additionally, a foundational Residual Atrous Spatial Pyramid Module in the separated flows enlarges the receptive field, allowing for the capture of multi-scale contextual information. Furthermore, a Modality-aware Dynamic Aggregation Module in the modality-complementary flow dynamically aggregates saliency-related cues from both modality-specific flows. Leveraging the proposed parallel triple-flow framework, we further refine saliency maps derived from different flows through a flow-cooperative fusion strategy, yielding a high-quality, full-resolution saliency map for the final prediction. To evaluate the robustness and stability of our approach, we collect a comprehensive RGB-T SOD benchmark, VT-IMAG, covering various real-world challenging scenarios. Extensive experiments on public benchmarks and our VT-IMAG dataset demonstrate that ConTriNet consistently outperforms state-of-the-art competitors in both common and challenging scenarios.
Via
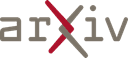